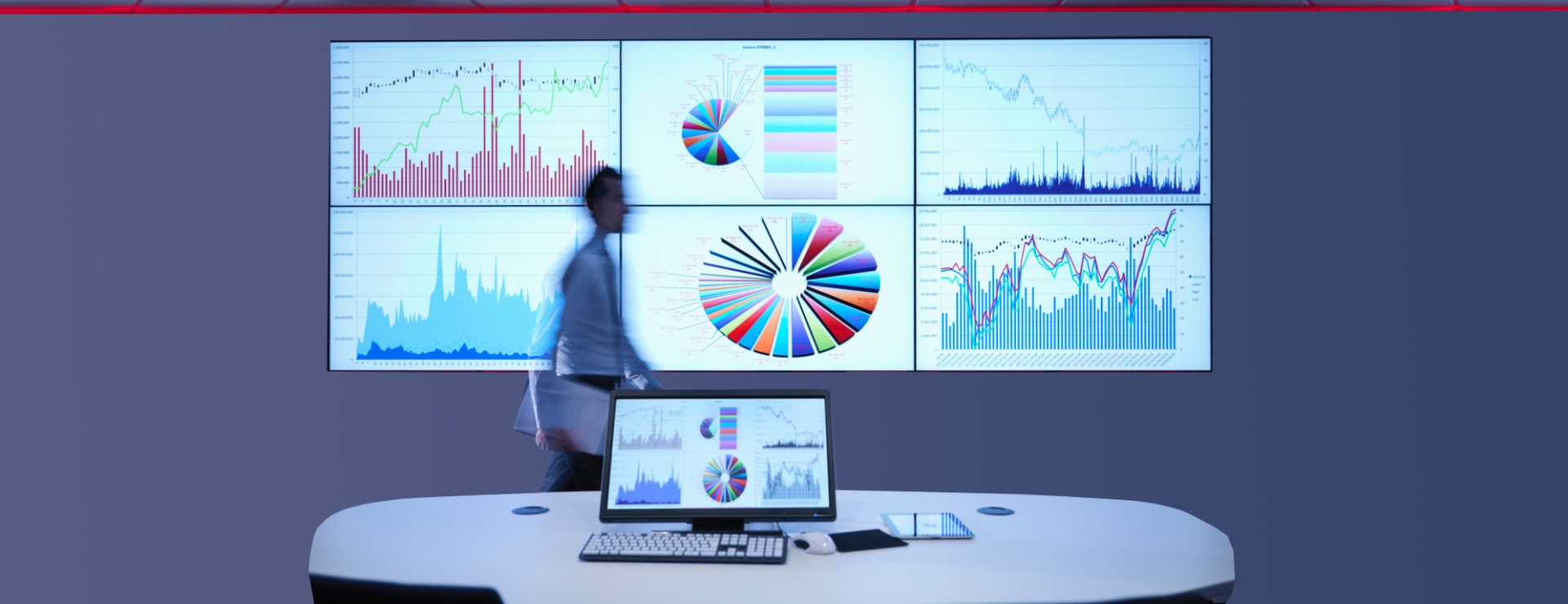
We are increasingly apprehensive about machines making unrestrained, unexplainable and potentially biased decisions that may impact our lives and wellbeing. Can we accept decisions when they aren’t reasonably understandable and appear unfair?
The easy answer is no. Should we, or can we, simply go back to the ‘good old days’ of people-powered decisions? It’s too late to put the algorithms back in a box. We’ve come to expect instantaneous, always available everyday services. The only practical way to deliver that at scale is with the help of algorithms. Instead, a better starting point may be to take time to recognise and correct any bias that was fed into the machines.
Data; the root of unfairness
To say that data is the only cause of unfairness is an over-simplification. A more complete explanation would be that algorithms are unfair because of the bias inherent in the systems that produce the data that taught the algorithms how to make decisions.
An algorithm is essentially a formula that explains the relationship between data and the thing you are trying to understand or predict. For example, that might be the likelihood that you will repay a loan or how you might respond to a request for a donation.
If the explanation comes from data, and that data was biased, then the explanation will be biased. Any data can result in unintentionally biased or unfair algorithms.
For example, prior to 2011 all car crash tests were conducted using dummies with an average male physiology. As a result, anyone with a different physiology, an average woman for example, was predicted to be more vulnerable to injury in a traffic accident. A bias in the system created a bias in the data which in turn resulted in faulty expectations based on gender.
A more recent example is facial recognition programs. These programs were initially less accurate at recognising non-white, non-male faces. The programs had been trained on predominately white male faces and so didn’t learn to identify other faces.
Some biases, such as those in the examples above, are relatively simple to identify and resolve. In practice, you will be dealing with data from systems that have been storing inputs and decisions for a long period of time and from many different processes and people. In the crash-test dummy example the data wasn’t biased. The underlying system that generated the data gave rise to a bias in the algorithm.
Bias is complex. The question becomes what type of bias you want to adjust for and how will you know you have succeeded.
Fair’s fair
Defining fairness sounds easy; it isn’t. Everyone has their own definition of fairness and what’s fair in one situation may not be fair in another.
We could adjust the weighting of a data set so that the proportion of 18-25-year-olds, for example, matches the proportion of 18-25 year-olds in the population. Is this fairer? Maybe. The answer might depend on the source we are using to estimate the proportion of 18-25 year-olds in the population and whether everyone will recognise it as the best source.
What about minorities or, for example, the proportion of Australians living in regional or rural areas relative to capital cities? If the representation of such segments is adjusted to match the population, that group will be under-represented in how the algorithm learns to make decisions.
In other situations, adjusting the training data set to more broadly represent the population may solve the problem of bias. In the facial recognition example, adjusting the sample so that it has an equal representation from the various groups would give the algorithm better training to recognise more people.
As such, recognising and adjusting for bias is not a simple task.
Change takes time
Increasing data and decision complexity means solutions will take time. Tools for assessing bias in data and models are just starting to become available. Even with clear definitions of fairness for given circumstances and ways to detect and counter bias, lasting change will take time.
Once we start removing bias from data and models then, over time, the systems will become less biased. We will need processes that monitor these changes so that, as the raw data becomes less biased, we are able to adapt the way we adjust for bias and create new algorithms using the less biased data.
Over time the systems for detecting and adjusting bias will themselves become more automated and dynamic.
Algorithms have the potential to make more consistently fair decisions than humans. However, to be helpful, these algorithms need good training. We need to decide what type of bias we are looking for and how to adjust for it in the data and modelling process. We need to continually check the outcomes and make further refinements. We need to develop transparent decision-making processes which provide individuals affected by a decision with the opportunity to question its fairness.
There will always remain a few questions that only people can answer; What is fair and what do we do about unfairness?
Related Posts
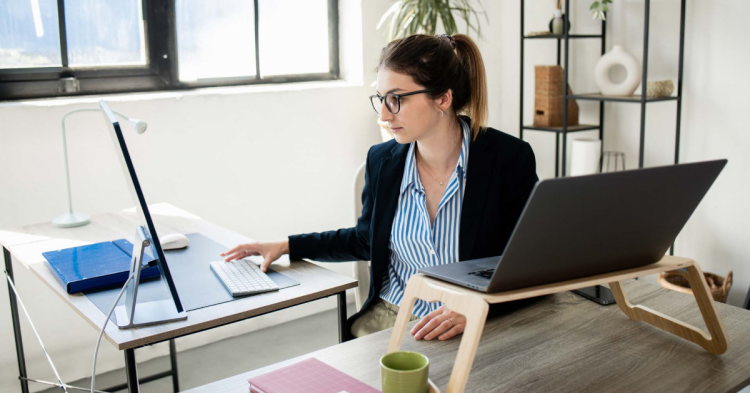
The Anti-Money Laundering and Counter-Terrorism Financing (AML/CTF) reforms are crucial for protecting the financial system from illegal activities. This overview explains the key changes brought about by Tranche 2 detailing essential compliance mandates and effective risk management practices.