Powering Smarter Decisions for Australia's SMEs
Smart decisions take many forms, but the best ones are usually those based on accurate and reliable analytics. The development of an Equifax solution for improving the accuracy and coverage of ANZSIC codes has improved the ability of SMEs to make decisions that can help increase profits and mitigate risk.
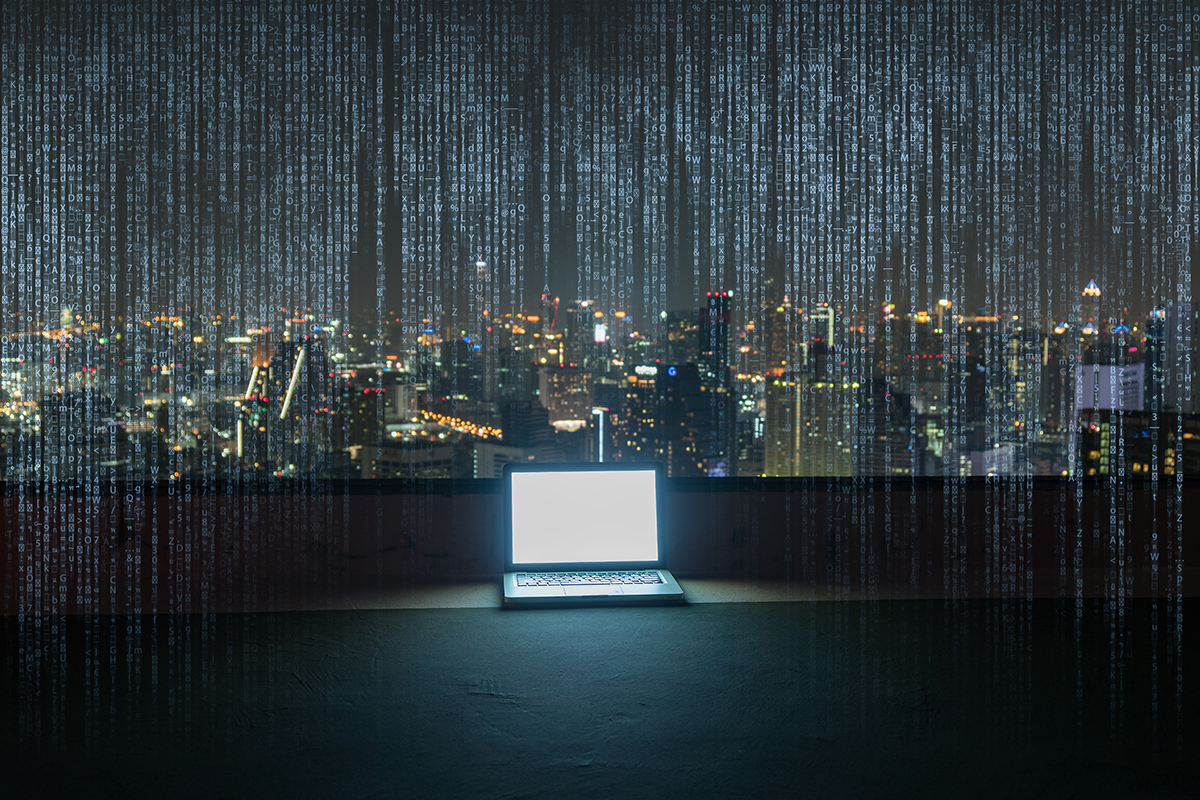
What are ANZSIC Codes?
The Australian and New Zealand Standard Industry Classification (ANZSIC) code was developed by the Australian Bureau of Statistics and Statistics New Zealand as a way to classify businesses into industries.
When a business applies for an Australian Business Number (ABN) through the Australian Business Registry, they must choose whichever ANZSIC code they believe best describes their core business. These industry codes are recognised as a crucial business level firmographic. Used alongside other descriptive attributes – such as the number of employees, revenue, year of establishment – they provide a clearer understanding of the customer and the market.
This in-depth view can assist SMEs to make better-informed decisions. For instance, if a customer is revealed as being from a high credit risk industry, this insight can be used to help insulate against potential risk. If a business is looking to improve their marketing and sales efforts, they can tailor their campaigns to specific audiences instead of taking a one-size-fits-all approach.
So, what's the problem?
The challenge is the unreliability of these industry codes. Companies are not compelled to update their ANZSIC code if their predominant activity changes over time. Consequently, the classification code listed for a business may be inaccurate if its core function has evolved.
Extrapolation has been an accepted way to cover the gap in known codes for many years. The difficulty has been to develop a model that is able to offer both widespread coverage of the millions of businesses in Australia and a high level of accuracy. Also, an approach capable of checking that each company has a code that matches its core activity.
The Equifax data and analytics team set out to find a solution that would maximise coverage with a high degree of accuracy through leveraging the latest predictive analytic capabilities.
Approach: Advanced Natural Language Processing on unstructured data
At Equifax, we sought to overcome previous technical hurdles through using technology sophisticated enough to process unstructured data. For example, the approach needed to be sophisticated enough to look at the title and from there, allocate the code that best represents what the business does.
We did this by using advanced natural language processing (NLP) techniques; we used advanced word embedding and word frequency analysis as well as applying advanced neural networks and deep learning. We then applied a unique ensemble modelling method to leverage predictions from multiple models and extract the optimal elements from each.
This approach gives Equifax access to a data set with codes assigned to each business and a confidence level that goes with the code. Customers can then choose to maximise either coverage or accuracy as best meets their need.
Outcome: A flexible solution to meet customer need
Businesses focused on improving their marketing efforts may want to opt for maximum coverage, which will vary with data selection criteria but can reach close to 100%. This is most suited for sales and marketing campaigns which aim to target a large number of prospects.
For businesses focussed on reducing credit risk, a trade-off can bemade by maximising the accuracy.
This will mean only ANZSICs that can be assigned with very high confidence are returned, accepting a potentially lower coverage. In this context, the business function is an attribute that contributes to the overall risk profile of the business, so accuracy is crucial. When a business has the confidence of knowing whether a customer or supplier is from a high or low-risk industry, they can make risk-based decisions such as whether to extend credit.
Contact us to find out more.
Related Posts
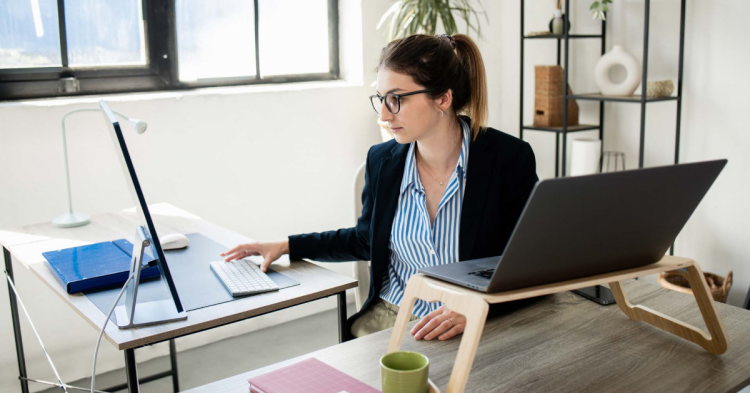
The Anti-Money Laundering and Counter-Terrorism Financing (AML/CTF) reforms are crucial for protecting the financial system from illegal activities. This overview explains the key changes brought about by Tranche 2 detailing essential compliance mandates and effective risk management practices.